Detection
Algorithm
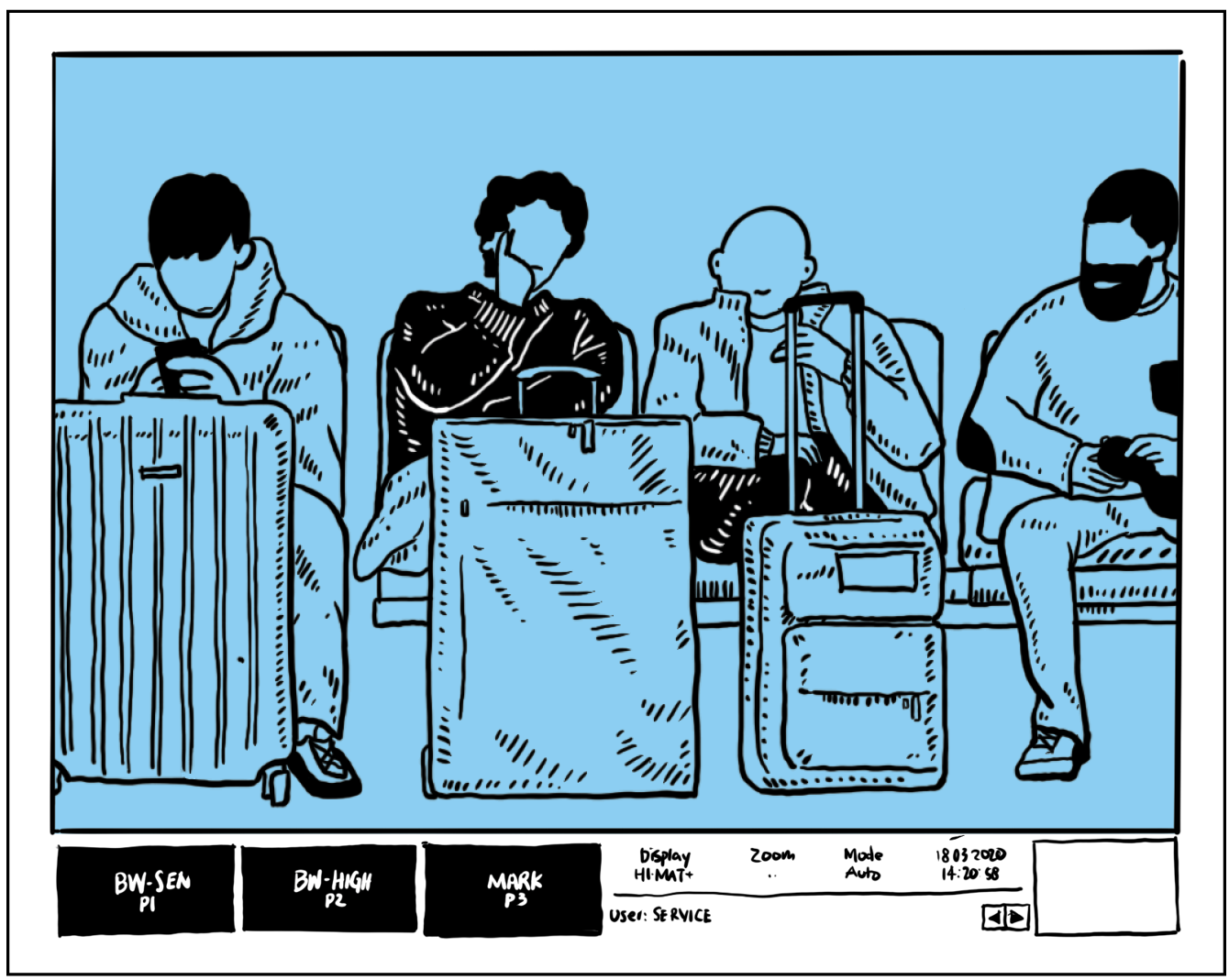
Detection algorithms combine CCTV footage with artificial intelligence to monitor deviant behavior and movement.
︎︎︎ Related entries:
Full Body Scanner
Passport
Non-Place
Safety
︎ Random Entry
Tags: systems design, security,
sorting, surveillance, technology
Full Body Scanner
Passport
Non-Place
Safety
︎ Random Entry
Tags: systems design, security,
sorting, surveillance, technology
Design Decisions
With ever expanding growth of the air travel industry, airport authorities needed a way to surveil passengers without having to examine each passenger rigorously. For this, three methods of sorting people have been developed to effectively sort the most probable threat to security from other passengers. These methods, each of which builds on the previous, are [1]:
1. Profiling,
2. Biometrics, and
3. Detection Algorithms
Profiling is the act of building up data about an individual into “profiles,” which are then compared against those of criminals to predict a person’s likely criminal or deviant behavior. Profiling relies upon vast quantities of information gathered about people and groups that are then shared, and involves sorting and identifying people by demographics (such as race, age, geographic origin, etc). In the US, this method was introduced following the TWA crash of 1996 [1].
Biometrics introduced a new and more granular method of sorting, ushered in by technological advances. It refers to the ability to track each person by identifying body parts, such as the iris, face, and palm signatures. In combination with profiling, biometrics allows the profiles stored about each individual to be summoned easily at checkpoints.
Detection algorithms, also known as artificial intelligence surveillance, is the latest and most technologically sophisticated of the three [2,3]. These algorithmic surveillance tools analyze real time CCTV and security camera footage, in order to look for and flag any potentially deviant or threatening movement among the passengers. To give an example, a technology currently widely used at US airports is called Exit Sentry, which monitors the movement of passengers walking through the exit corridor of secured areas of the terminal. A passenger walking the wrong way, or trying to enter a secured area is warned with a flashing light. If the person persists, a siren alerts security staff [1].
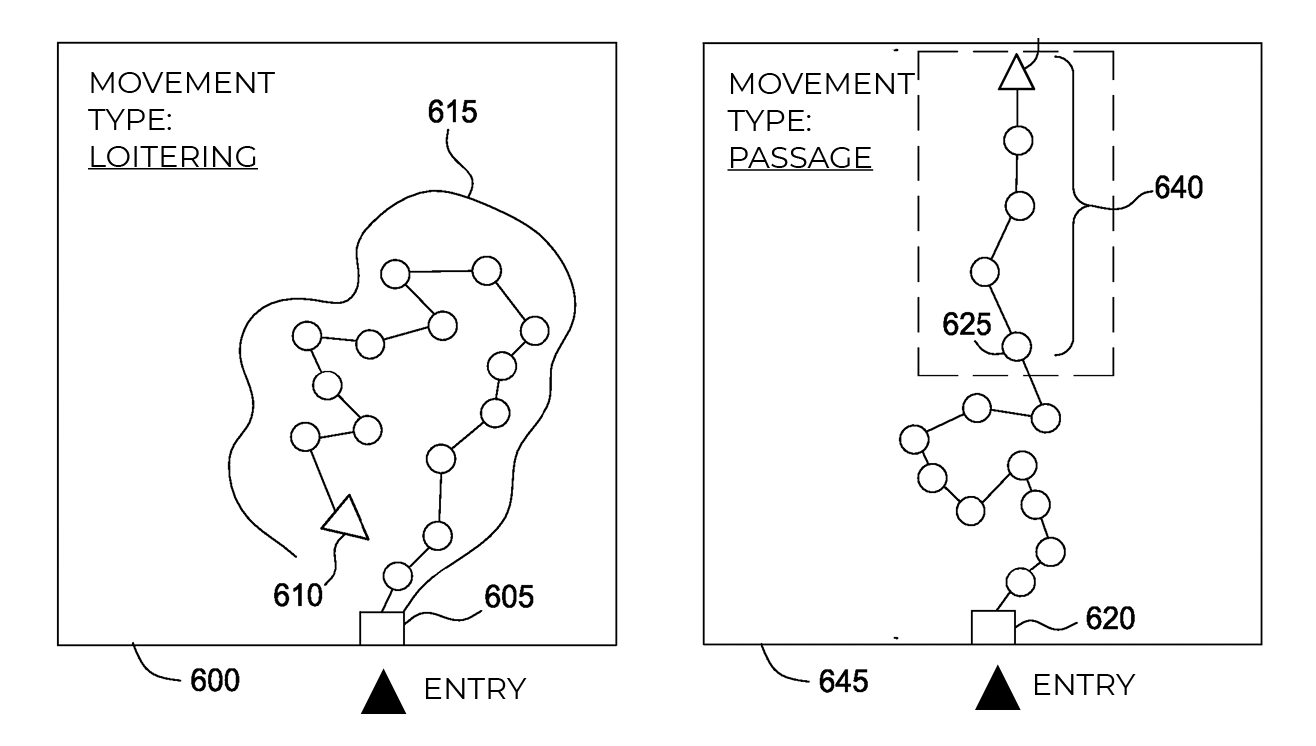
Algorithmic surveillance can be understood as a way of identifying the intent to do harm, rather than identifying the capacity to do harm. These technologies focus on monitoring the movement of people as suspicious or potentially deviant. In contrast, methods that identify the capacity to do harm include X-rays and Full Body Scanners.
These three methods of sorting people build upon each other and are used in combination. For example, detection algorithms add additional information to the pool of data collected for biometric surveillance, such as gait recognition, which seeks to identify the identity of individuals by their distinctive walking styles. Or, a passenger flagged by the detection algorithm could be detained by security staff, biometrically identified by fingerprint, and have their profile summoned in order to assess the level of security risk.
Effects on Passengers
Detection algorithms could be seen as both potentially more convenient for certain passengers, and more concerning for passengers’ civil liberties.
This surveillance method lessens the pressures at security checkpoints on both passengers and staff, as it makes surveillance a less visible presence in the airport. It is possible that further development of this technology could help erase the spatial chokepoints in the terminal, and allow for more expedited passage through the airport [4].
However, it also raises a slate of civil liberties concerns [5]. Although it is well documented that biases and prejudice can significantly affect algorithmic systems through its human developers and users [6, 7], algorithms are often seen as ‘impartial’. For certain populations, such as Arabs, Muslims, and Latinos in the US, detection algorithms can be seen as a “license to harass” [8]. There is a risk that human developers inadvertently teach algorithms to flag mannerisms and behaviors more prevalent in minority groups as ‘suspicious’, and that those detection algorithms would further perpetuate harmful racial or religious profiling.
Additionally, significant scientific research has undermined the premise that behavioral cues can indicate deception at all: “Liars do not seem to show clear patterns of nervous behaviors such as gaze aversion and fidgeting… People who are motivated to be believed look deceptive whether or not they are lying” [6]. The available research indicates that sorting potentially dangerous individuals through behavior observation is little more reliable than blind guesswork.
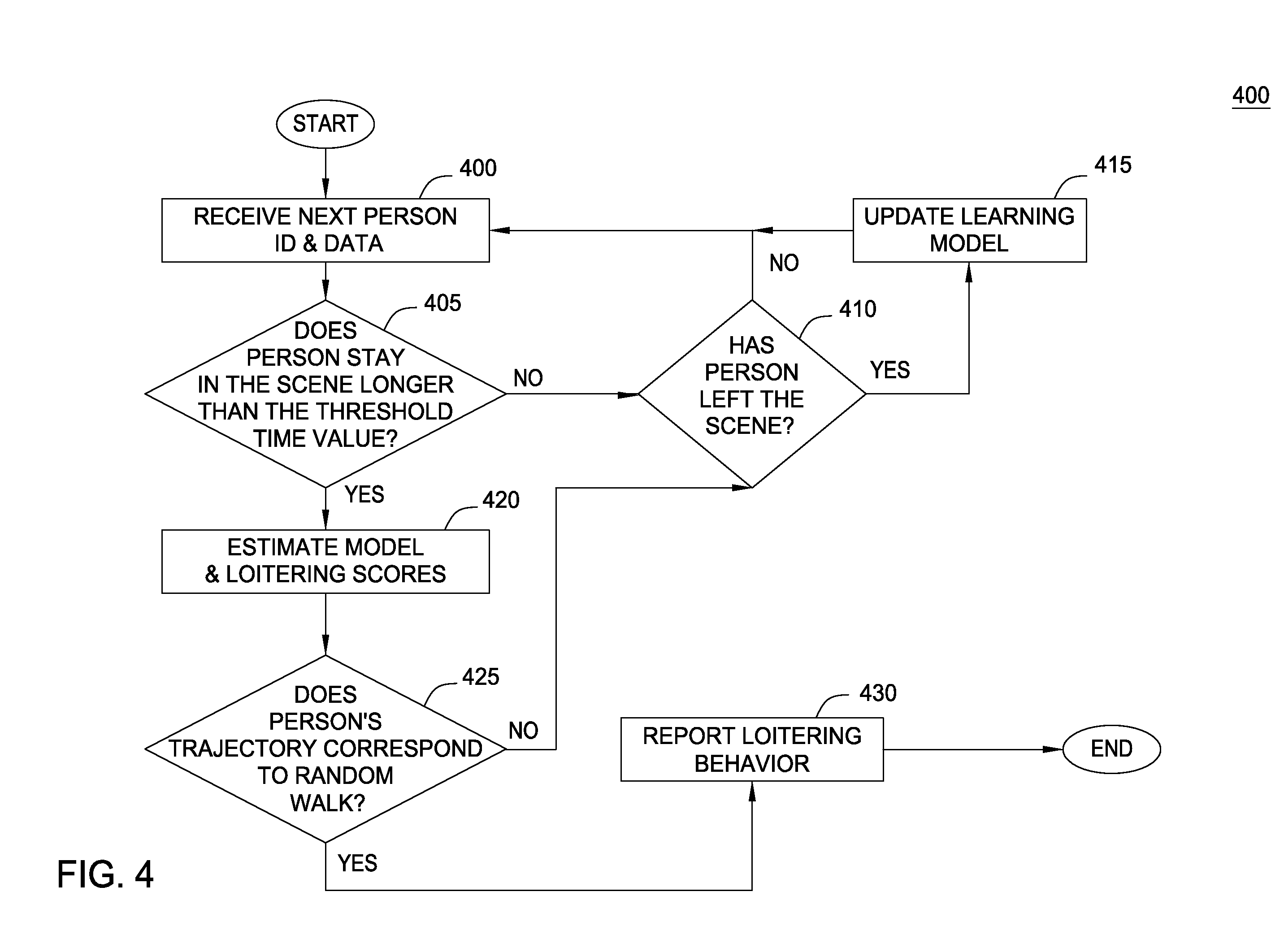
Examples criteria used by the surveillance algorithm software to track loitering and movement.
- Adey, Peter. 2003. “Secured and Sorted Mobilities: Examples from the Airport.” Surveillance & Society 1 (4).
-
Norris, C. (2002) “From personal to digital: CCTV, the panoptican, and the technological mediation of suspicion of social control”. In. D. Lyon (ed.) Surveillance as Social Sorting: Privacy, Risk, and Digital Discrimination. London and New York: Routledge, 249-281.
-
Graham, S. and D. Wood (2003) “Digitising surveillance: categorisation, space, inequality”. Critical Social Policy, 23(2): 227-248.
- Arup. 2016. “Future of Air Travel.” Arup & Partners, Intel Corporation.
- VISCUSI, W. KIP, and RICHARD J. ZECKHAUSER. 2003. “Sacrificing Civil Liberties to Reduce Terrorism Risks.” Journal of Risk and Uncertainty 26 (2/3): 99–120.
-
Crawford, Kate. “Trouble with Bias.” Keynote Lecture, Neural Information Processing Systems, 2017.
-
Hamidi, Foad, Morgan Klaus Scheuerman, and Stacy M. Branham. 2018. “Gender Recognition or Gender Reductionism?: The Social Implications of Embedded Gender Recognition Systems.” In Proceedings of the 2018 CHI Conference on Human Factors in Computing Systems - CHI ’18, 1–13. Montreal QC, Canada: ACM Press.
- Ackerman, Spencer. 2017. “TSA Screening Program Risks Racial Profiling amid Shaky Science – Study.” The Guardian, February 8, 2017.